Dark matter and dark energy have been the subject of study for cosmologists and physicists who are striving the understand the world around us in its entirety. The composition of the universe is an age-old inquiry that these researchers have probed into. And while we do have estimates of the likely percentages of baryonic matter, dark matter, and dark energy at 5%, 27% and 68%, respectively, researchers have been trying to improve these estimates and optimize the computational expense of the statistical methods employed to analyze cosmological data.
One such paper was released recently by a team of researchers hailing from ETH Zurich. In the paper, titled "Cosmological constraints with deep learning from KiDS-450 weak lensing maps", the team of researchers detailed their method to study dark matter in the cosmos by employing convolutional neural networks (via Nvidia Developer News Center).
The team began by first training the convolutional neural network (CNN) using Nvidia P100 GPUs on the data from a computer-generated simulation of the universe. With this, the model was able to learn the various hidden features and the weights associated with the model to improve its accuracy. Subsequently, the trained model was then put to test with the KiDS-450 tomographic weak lensing dataset, which contains the shapes of approximately 15 million galaxies.
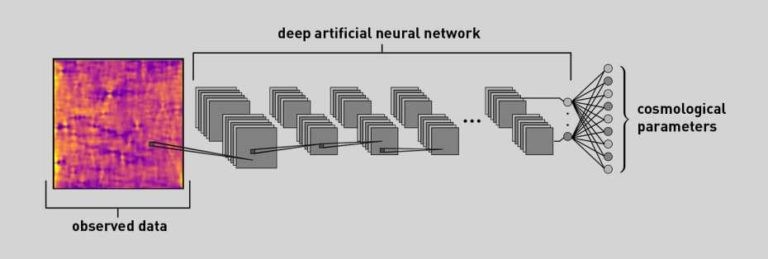
In the results, the researchers found that the deep learning-based model performed better than traditional methods of inference. Specifically, the former delivered 30% more accurate values than those made by scientists using traditional statistical methods. In addition, the model was also faster than using the Hubble telescope with the team saying that twice as much time would have been spent in gathering data alone from the telescope for the experiment.
A Ph.D. student at ETH Zurich and the lead author of the study, Janis Fluri, commented on the team's work saying that it was an industry-first and that it allowed the extraction of more information from the data analyzed:
"This is the first time such machine learning tools have been used in this context. We found that the deep artificial neural network enables us to extract more information from the data than previous approaches. We believe that this usage of machine learning in cosmology will have many future applications.”
While in the abstract of the paper, the team claims that the technique is a promising prospect for cosmological data analysis in the future:
We compare this result to the power spectrum analysis on the same maps and likelihood pipeline and find an improvement of about 30% for the CNN. We discuss how our results offer excellent prospects for the use of deep learning in future cosmological data analysis.
For more information, you can study the paper published here.