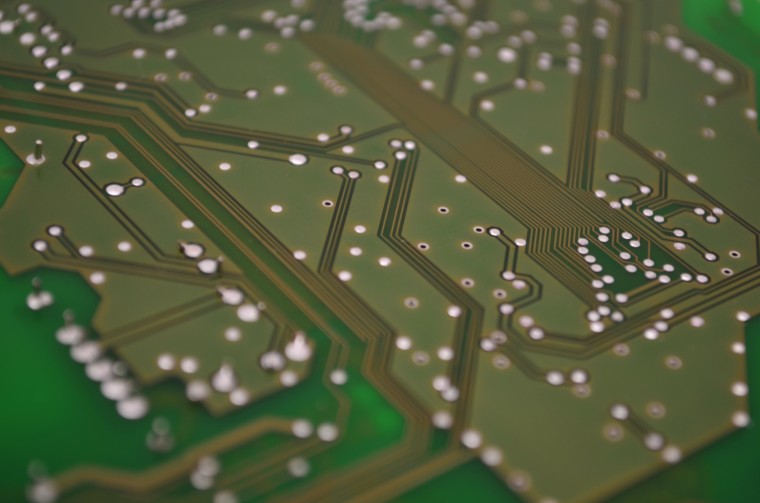
Silicon Valley-based startup Mipsology announced today that its Zebra AI inference accelerator achieved the highest efficiency based on the MLPerf inference test. The benchmark, which measures training and inference performance of ML hardware, software, and services, pitted Mipsology's FPGA-based Zebra AI accelerator against venerable data center GPUs like the Nvidia A100, V100, and T4. Comparisons were also drawn with AWS Inferentia, Groq, Google TPUv3, and others.
The results above show Zebra running on the Xilinx Alveo U250, U200, U50LV, and ZU7EV accelerator cards. The efficiency of computation in FPS/TOPS of the ResNet 50 architecture was significantly higher on Zebra, with the Xilinx Alveo U250 achieving more than 2x higher peak performance efficiency compared to all other commercial accelerators. Ludovic Larzul, CEO and founder of Mipsology, added that these results are from MLPerf's 'closed' category, which is a rigorous test that requires the same model and optimizer as the reference implementation for drawing comparisons:
“We are very proud that our architecture proved to be the most efficient for computing neural networks out of all the existing solutions tested, and in MLPerf’s ‘closed’ category which has the highest requirements. We beat behemoths like NVIDIA, Google, AWS, and Alibaba, and extremely well-funded startups like Groq, without having to design a specific chip and by tapping the power of FPGA reprogrammable logic.
The results show the computational advantages of specialized FPGA-based technologies, which are gradually making their way into the market. They also add to the critique of TOPS (Tera Operations per Second) being a direct indicator of computational performance. With a peak TOPS of 38.3, the Zebra-powered Alveo U250 accelerator card significantly outperformed competitors in terms of throughput per TOPS, exhibiting performance close to a Tesla T4 on the MLPerf v0.7 inference results, despite having 3.5x fewer TOPS.
"Perhaps the industry needs to stop over-relying on only increasing peak TOPS. What is the point of huge, expensive silicon with 400+ TOPS if nobody can use the majority of it?”, questioned Larzul, pointing to the diminishing returns of pumping in more TOPS on the foundations of contemporary circuitry.
8 Comments - Add comment